Outcome Homogenization
Written by
Tiffany ClarkAbout the Author
Tiffany is an experienced workforce development expert with extensive experience in the public sector, course development, and online learning.
Reviewed by
VidCruiter Editorial TeamAbout the Reviewer
The VidCruiter Editorial Team offers practical recruitment advice, drawing on insights from seasoned professionals. Our award-winning content, supported by industry specialists, underscores VidCruiter's dedication to innovation in recruitment.
Last Modified
Apr 17, 2024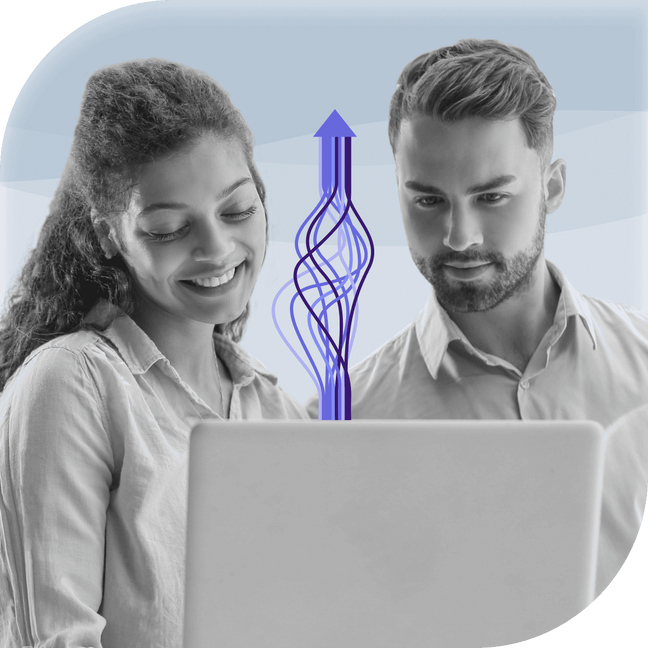
In the context of artificial intelligence (AI), outcome homogenization refers to the tendency of AI models and systems to produce outcomes that are overly similar or uniform across various scenarios. This issue is particularly relevant in machine learning, where algorithms use data for making predictions.
Because of the mathematical nature of algorithms that focus on efficiency, outcome homogenization can cause a loss of solution diversity. This occurs because AI models can often overlook creative alternatives, favoring similar solutions even for different problems.
AI models are more at risk of outcome homogenization when the training data lacks diversity or contains biases. As a result, these models fail to adequately represent or function well across diverse situations.
Outcome homogenization has significant ethical and societal implications, particularly in areas like credit scoring, recruitment, and law enforcement. It can lead to discrimination, unfairness, and lack of representation of marginalized groups.
To address these concerns, there is a growing emphasis on working to incorporate diversity and inclusion in AI systems. The process for improving AI systems in this way includes developing algorithms that are more sensitive to a range of demographics and contexts.
Example of Outcome Homogenization:
AI algorithms used in HR are usually trained on historical data. This includes employee performance records and resumes provided by hired candidates. In many cases, the data may reflect non-diverse hiring practices or historically concerning biases. When this occurs, the AI system can easily learn to replicate the patterns in these sets of data. The result could be a company hiring people with specific backgrounds or candidates who went to certain schools, thereby prioritizing similar candidates and excluding marginalized groups.
Another example is an AI algorithm creating a narrow definition of the supposed ideal candidate. Because AI systems are programmed to identify the ideal candidate, factors such as specific skills and educational backgrounds can narrow down the candidate pool. When this happens, desirable characteristics such as transferable skills or diverse experiences are often ignored.
Related Terms
Algorithmic Bias
refers to AI tending to favor certain groups over others systematically because of a lack of diversity and biases present in training data sets.
Data Bias
involves biases inherently present in data sets that are used to train AI models. Historical data sometimes lacks diversity or reflects certain prejudices, causing the AI system to replicate the biases when making decisions.
Unconscious Bias
refers to biases of which humans or AI models are unaware. If not properly checked and controlled, AI models can amplify unconscious biases.
Skill-Based Hiring
is the approach by which hiring focuses solely on the abilities, knowledge, and skills of job candidates instead of traditional factors such as previous job experience or education levels. The problem with AI skills-based hiring is that the models don’t take into account diversity, equity, and inclusion.