AI Hallucination
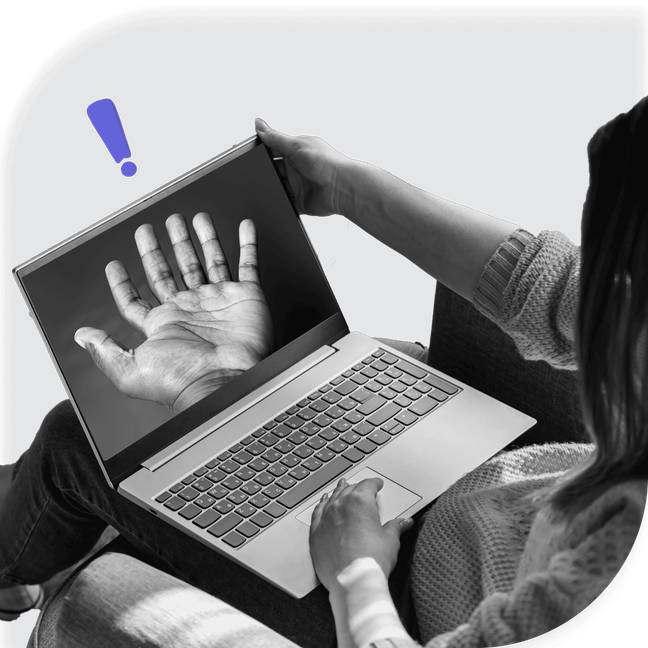
An AI hallucination occurs when AI models present incorrect or misleading information as if it is a fact. The term AI hallucination derives from the hallucination phenomenon in human psychology. In the field of psychology, a hallucination is defined as a false perception of events or objects that involve the human senses of taste, smell, touch, sound, or sight. In simple terms, someone who is hallucinating can not tell the difference between their hallucination and reality.
While human hallucination is typically associated with false perceptions, AI hallucinations refer to the generation of incorrect or unjustified information or responses. While AI tools are trained to generate answers to a user’s query, these models generally lack the reasoning to apply contextual logic. Additionally, the majority of current AI models are unable to consider factual inconsistencies. In short, AI models are trained to satisfy the user's request and will sometimes generate false information in this pursuit.
Causes of AI Hallucination Include
- Overfitting: AI models are trained on limited data sets and are unable to generalize new data effectively.
- Low-quality, insufficient, or outdated training data: When an AI model misunderstands a prompt, it will rely on its limited data set and generate an inaccurate response.
- Adversarial attacks: Users may deliberately input prompts designed to confuse AI models.
- Use of slang expressions or idioms: Many AI models are not trained in current slang words or idioms. This can lead to outputs that don’t make sense.
Example of AI Hallucination
Consider an employee who is working on a high-priority project that requires them to work at irregular times of day so they can communicate with a team in a different timezone. If an AI model is trained to flag potential burnout risk, it may incorrectly categorize the employee's late-night work hours as an indicator of overworking. Then, the AI model generates a report to HR suggesting the employee is at risk of burnout. When this occurs, HR might inappropriately intervene, which can create confusion in the company or with the employee.
Related Terms
Model Bias
occurs when an AI model generates systematically prejudiced outputs. This usually occurs when the algorithms or training data is biased. Model bias isn’t specifically the same as hallucinations, but it can contribute to inaccurate content generation.
Generalization Error
is a term that describes the discrepancy that occurs between an AI model’s performance on training data versus new data. High generalization errors often manifest in inaccurate outputs when models encounter new input.
Data Drift
occurs when input data is distributed over time, which can sometimes result in changes to the data. With data drift, the performance of the model may degrade, leading to situations where the model begins to produce less accurate output.
Out-of-Distribution Data
is a term that refers to a model encountering data that is significantly different from the model’s training data. This can produce incorrect output that is similar to AI hallucinations.
Confabulation
is a term borrowed from the field of psychology. This term refers to how an AI model can unconsciously create distorted or completely fabricated memories about itself.
Anomaly Detection
identifies unusual data patterns. Anomaly detection can often be used to flag AI hallucinations.
Error Analysis
is the process of examining incorrect outputs. This process can help models improve by understanding the root cause of the errors.
Robustness and Reliability
are terms that refer to a model’s stability in various conditions. When a model lacks robustness and/or reliability, it can lead to incorrect outputs that include AI hallucinations.