Inference in AI
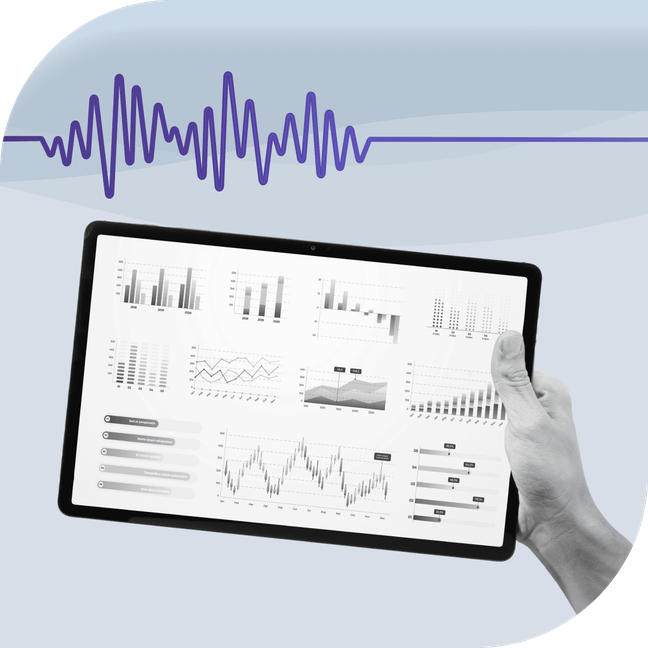
Inference in AI refers to the process in which a previously trained model makes decisions or predictions based on new or previously unseen input data. AI inference uses an “inference engine” to apply logical rules to a knowledge base. While machine learning focuses on training models to make predictions and recognize patterns, AI inference is the subsequent step, where models process new data. Training a model is a one-time investment, where AI inference is ongoing.
The AI inference process resembles the decision-making of a well-trained and knowledgeable human expert, drawing on their training, education, or wealth of experience. However, AI inference operates at a scale and speed unattainable by humans, making it an invaluable tool for tasks requiring accurate and rapid decision-making.
Without AI inference, machines cannot learn from new data. Most importantly, AI inference can make decisions in real time, reducing latency and improving system responsiveness. This capability establishes AI inference as a driving force of innovation across business and industry applications.
Examples of AI Inference
AI Inference in the Hiring Process
AI inference can streamline interview scheduling by coordinating the availability of candidates and interviewers. It can help compile lists of appropriate interview panelists, suggest optimal interview times, send out calendar invites, distribute interview materials, and even reschedule if conflicts arise, all without human intervention.
AI Inference with Established Employees
After hiring, AI inference can analyze new employee performance and adaptation to predict early signs of employee turnover or identify areas for development. By identifying these signs early, organizations can proactively address the underlying issues, potentially improving employee satisfaction and retention rates.
Related Terms
Model Inference
is the process by which deep learning or trained machine learning models apply what it has learned to new data. For example, in a neural network that has been trained to recognize images, inference is the process of feeding new images into the network to obtain a prediction.
Inference Engine
Is the term used for the component that deduces new information by applying logical rules to a knowledge base.
Inference Phase
refers to the contrast to the training phase in machine learning. When undergoing training, the machine learning model adjusts its parameters to learn from a data set. In the inference phase, the trained model makes analyses or predictions.
Statistical Inference
refers to how a model draws conclusions about the characteristics of a population based on a sample.
Hardware Inference
is the specialized hardware used to run AI models efficiently for inference. This can include TPUs, GPUs, and dedicated AI accelerators that have been optimized for efficient processing.