Classification Bias
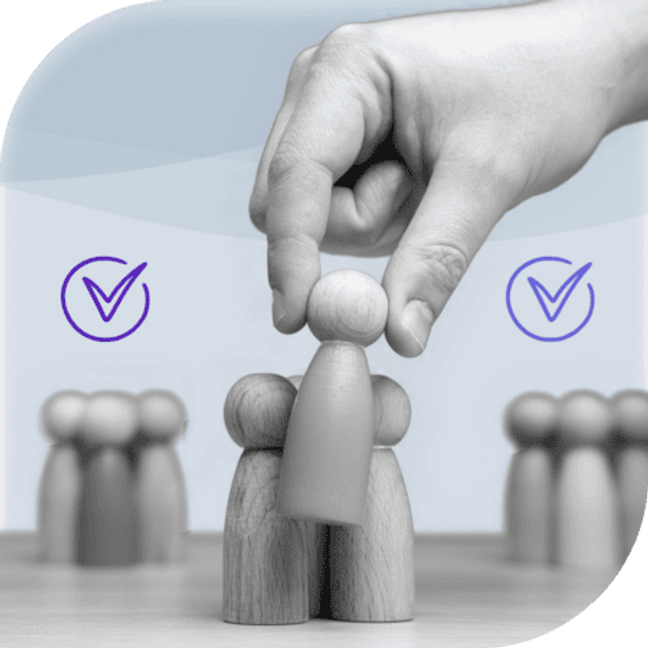
Classification bias occurs when data algorithms rely on unrepresentative, biased, or inaccurate data to systematically undermine historically disadvantaged groups, including women, and racial and ethnic minorities.
The term classification bias highlights the risk that algorithms can score workers and sort them in ways that make disadvantages and inequality worse in terms of sex, race, or other protected characteristics. When data-driven automated decisions control access to employment opportunities, the end results are similar to some of the systematic patterns of disadvantage that initially spurred antidiscrimination laws.
A 2017 paper published in the William & Mary Law Review titled “Data-Driven Discrimination at Work” (abstract) outlines how existing employment discrimination laws must be adapted to meet the challenges of algorithmic decision-making. The researchers explain that employers increasingly rely on algorithms to make decisions about which candidates get interviewed, hired, and promoted.
According to the authors of the William & Mary Law Review paper (full paper), a close reading of the statutory text of Title VII of the Civil Rights Act of 1964 suggests that classification bias is directly prohibited by law. However, there is currently no legal response to data-driven discrimination practices such as classification bias.
An organization’s data analytics tool can be used to make personnel decisions by using granular data about the behavior of employees or candidates both in and out of the workplace. The widely accepted thinking is that these technologies can help employers or recruiters recruit more talented workers by screening for an applicant pool’s most eligible candidates.
Proponents of data-driven hiring practices believe that the data can predict a person’s likelihood of success at a specific job and that the supposedly neutral data reduces the risk of biased human decisions. However, algorithms can discriminate because data is not neutral.
Example:
Data models do not rely on indications such as on-the-job experience or formal education. Rather, third-party aggregation tools harvest candidate information from the internet, including social networking data, the number of contacts someone has, how frequently they post on social media, their likes and preferences, and who follows them. With these types of tools, employers can also gather off-duty behavior patterns of both candidates and current employees.
One example is that data miners can determine if a woman is pregnant, what people eat, how they spend their free time, and how frequently they exercise. Algorithms are trained and developed to screen, evaluate, and score candidates for particular positions.
Related Terms
Cognitive Bias
Confirmation Bias
refers to a person’s tendency to consciously or subconsciously seek information that confirms their opinions or views while disregarding input that challenges their perception.