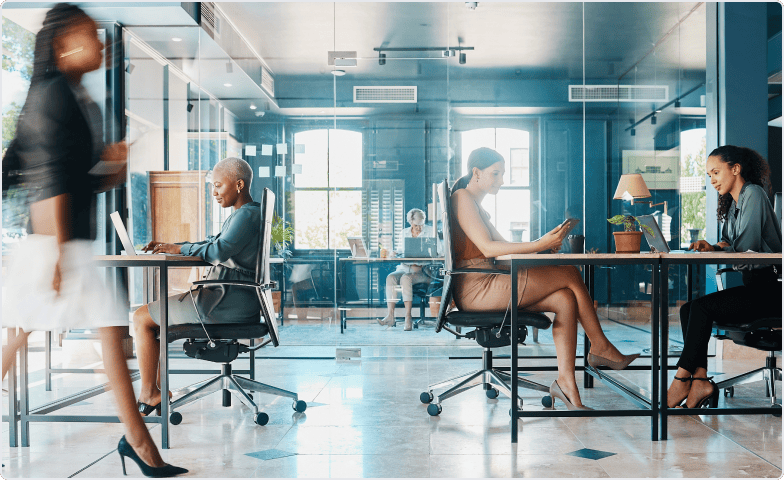
How to Create a Framework for AI-Augmented Recruiting
Learn what you need to know to draft and implement an AI governance policy for your hiring.
Artificial intelligence (AI) bias in hiring occurs when an AI system shows a preference or prejudice toward certain candidates. Computer systems lack human emotions and experiences, but they can still perpetuate biases from their training data or how they were built. They can also misinterpret data, which could lead to biased predictions.
AI hiring bias occurs when an AI model unfairly or inaccurately favors or disapproves of certain candidates. This bias can lead AI tools to reject qualified candidates for reasons that are completely unrelated to job performance, such as race or gender.
Here are the four most common types of AI hiring bias.
Algorithmic Bias. Algorithmic bias occurs when errors in an AI model’s algorithm lead it to make unfair or inaccurate decisions. For example, if an AI model started favoring candidates with names that start with the letter J or those who played baseball over basketball.
Sample or Representation Bias. This bias happens when an AI model’s training data is not diverse enough and over or underrepresents specific populations. To illustrate, an AI system trained primarily on male candidate data may start to show prejudice against female candidates.
Predictive Bias. This bias occurs when an AI system consistently overestimates or underestimates a particular group’s future performance. An example would be if an AI tool consistently ranks candidates from one school lower than those from other institutions despite having similar backgrounds or levels of experience.
Measurement Bias. This bias occurs when errors in an AI model’s training data set lead it to make inaccurate or unfair conclusions when working with real data. For example, if you train an AI system on incorrect data from your company’s top performers, it won’t accurately identify the right traits in candidates.
Do job seekers trust AI to be unbiased?
According to a 2023 American Staffing Association Workforce Monitor report, nearly half of employed US job seekers (49%) believe AI recruitment tools are more biased than their human counterparts.
AI systems can inherit the biases of the humans who make and train them, as well as develop their own biases. Here are three key causes of AI hiring bias.
An AI tool’s training data needs to accurately reflect the population in which it will be used. If this dataset is too small, lacks diversity, or is missing details, this can lead to bias. For example, research from Sony found that image datasets overrepresent people with lighter, redder skin tones, and underrepresent darker, yellower skin, leading to inaccuracies like classifying people with redder skin hues as “more smiley.”
The engineers who develop AI algorithms can unintentionally incorporate their biases into the software, based on how they label the training data or the characteristics they choose the tool should focus on. An example of this would be if an AI recruitment tool’s training data has categories for education, occupation, and gender, the tool may consider gender as an equal contributor to success, which could lead to discrimination.
AI tools can ‘hallucinate’ responses and produce outputs that are not based on training data or follow any identifiable pattern. For example, in 2022, Meta’s Large Language Model (LLM) Galactica made up wiki articles and fake papers, sometimes attributed to real authors. In a hiring context, this could look like an AI tool appearing to shortlist candidates based on relevant factors, when in reality, it is picking people based on made-up attributes.
Unchecked bias in AI tools can lead to incredibly harmful effects—most significantly, hurting organizations’ efforts to become more diverse, equitable, and inclusive workplaces.
AI hiring bias can lead AI systems to reject qualified candidates based on factors that would not actually impact their performance. For example, in 2020, a UK-based makeup artist lost her job after an AI screening program negatively scored her body language despite performing well in skills evaluation. With more organizations using AI in recruiting, AI-rejected candidates might not only miss out on one job but could be excluded from their entire industry or the job market altogether.
If an organization lacks diversity, and it trains an AI model based on past employee data, it could potentially encode hiring biases into the system. As a result, candidates from historically underrepresented or discriminated groups may get ranked lower or rejected because they are too dissimilar from past employees.
Sometimes, the best employees have non-traditional backgrounds or career paths. These types of candidates bring a unique perspective that can boost innovation within an organization. However, AI models trained on past and current candidate data will look for candidates that are most similar to the people who are already in your organization, which could lead you to miss out on these unique applicants.
What are the real-life implications of AI bias in hiring? Here are two notable incidents that made headlines.
Candidate accessories and video background impacted hiring assessment scores
In 2021, a team of German journalists tested an AI video interview platform and found that wearing different accessories, hairstyles, and outfits impacted a candidate’s personality score. The same experiment also found that factors like having a picture or a bookshelf in the background or changing the brightness or saturation of a video could impact assessment results.
Amazon’s gender-biased hiring algorithm
In 2014, Amazon created a machine-learning tool to rate job applicants. However, since it was trained on company resumes that were mostly from men, the tool started to show bias toward male candidates. It penalized resumes with the word ‘women’ and downgraded graduates from all-women’s colleges. Amazon tried to fix the problem but eventually disbanded the team behind the tool in 2017.
AI hiring bias can lead to recruitment decisions that aren’t just unfair, but could also put your organization in ethical and legal hot water.
The most significant ethical issue with AI hiring bias is that it leads to unfair hiring decisions. It takes an incredible amount of work to apply and interview for a job, and it’s unethical for candidates to be rejected or down ranked due to superficial factors like wearing glasses or a headscarf.
Even AI experts don't completely understand how AI systems make certain decisions. Therefore, relying on them to make hiring decisions that significantly impact people’s lives is problematic at best and irresponsible at worst. This lack of transparency also makes it challenging for workplaces that use AI tools to identify and fix bias issues in their platforms.
If a candidate feels they have been treated unfairly by an AI system during the hiring process, they could sue your organization for AI discrimination. Additionally, more governments and regulatory bodies are creating laws and restrictions to control the use of AI in hiring. If your organization is found to be guilty of discrimination or non-compliance, it could lead to costly legal battles, fines, and reputational harm.
For example, the General Data Protection Regulation (GDPR) in the European Union has guidelines on automated decision-making that could apply to AI hiring tools.
Even if we can’t completely eradicate bias from AI systems, there are important steps organizations can take to mitigate its impact and create fairer and more equitable hiring processes.
Does your AI training set accurately represent the population in which you’re working? How does AI output compare to human-led hiring decisions? Regularly auditing your AI system, both internally with a diverse team of stakeholders and externally by a third party, will help you spot and address biases and optimize your system over time.
Autonomous testing, which involves using techniques like oversampling to address inaccuracies caused by incomplete past data, can also spot biases in datasets. For example, MIT researchers developed an AI system called DB-VEA, which automatically reduces bias by re-sampling data.
New York City now requires employers to conduct annual AI bias audits.
The law, which took effect in July 2023, mandates that New York City employers conduct yearly third-party AI “bias audits” on technology platforms used for hiring or promotions to ensure these AI tools don’t discriminate based on race or gender. They must also publish audit findings on their websites.
Artificial intelligence technologies are only as good as the data used to train them. Using more extensive and diverse training datasets that include people of different cultures, races, ages, religions, sex and gender identities, sexual orientations, and abilities can help mitigate the impact of bias.
Integrating big and small data into training sets can also enhance AI’s accuracy. Big data analysis tends to emphasize correlations, which can result in bias if the AI tool incorrectly interprets a correlation as a causal relationship. Small data is more user-specific and offers crucial details that could help an AI tool avoid these mistakes.
By way of example, an AI tool may incorrectly assume that candidates attending prestigious universities are more likely to succeed because many successful employees have such backgrounds. However, having access to more specific information about each candidate, like their individual experiences, skills, and achievements, could help AI see beyond these broad patterns and make better decisions.
Our belief is that all hiring decisions should be human-led, but AI can help address the impact of bias throughout the interview process by providing feedback and guidance to your recruitment team. For example, interview intelligence software can help hiring managers select a diverse group of professionals for a panel interview or help interviewers maintain consistency by providing feedback on talk times and interruption rates.
In the same way, you would not let an intern make CEO-level decisions, an AI tool should not make hiring decisions unchecked. A human should always be involved in the process to catch any bias issues that the technology might miss.
AI is a powerful recruitment tool that can sort through and analyze vast amounts of data in seconds and exponentially speed up hiring processes. However, it’s not a magic fix. Recruitment professionals need to be aware and cautious of its limitations, particularly around bias.
By regularly reviewing AI systems and using them in a supporting role for human-led decision-making, we can mitigate the impact of AI hiring bias, while still taking advantage of its time and resource-saving benefits.
AI bias in hiring occurs when an AI system favors candidates with certain characteristics over others. For example, an organization’s AI screening tool was found to be biased against older applicants when a candidate that had been rejected landed an interview after resubmitting their application with a different birthdate to make themselves appear younger.
AI can help mitigate the impact of bias, but it cannot remove it altogether. If an AI system is trained on biased data or is created by a biased engineer, it could perpetuate and even amplify these prejudices. To tackle hiring bias, humans need to regularly monitor AI decisions and refine their algorithms to ensure fairness. In turn, AI technology can help mitigate the impact of human bias in hiring by developing structured interviewing questions and automating processes to improve consistency.
It’s difficult to completely eliminate AI bias, but we can take steps to reduce its impact. Organizations should conduct regular AI bias audits to ensure fairness and use larger and more diverse datasets to train them. Creating more diverse, equitable, and inclusive AI development and audit teams can also help mitigate bias by bringing different perspectives and experiences to the table.
Modernize your hiring process with expert insights and advice.